Microbial load can influence disease associations
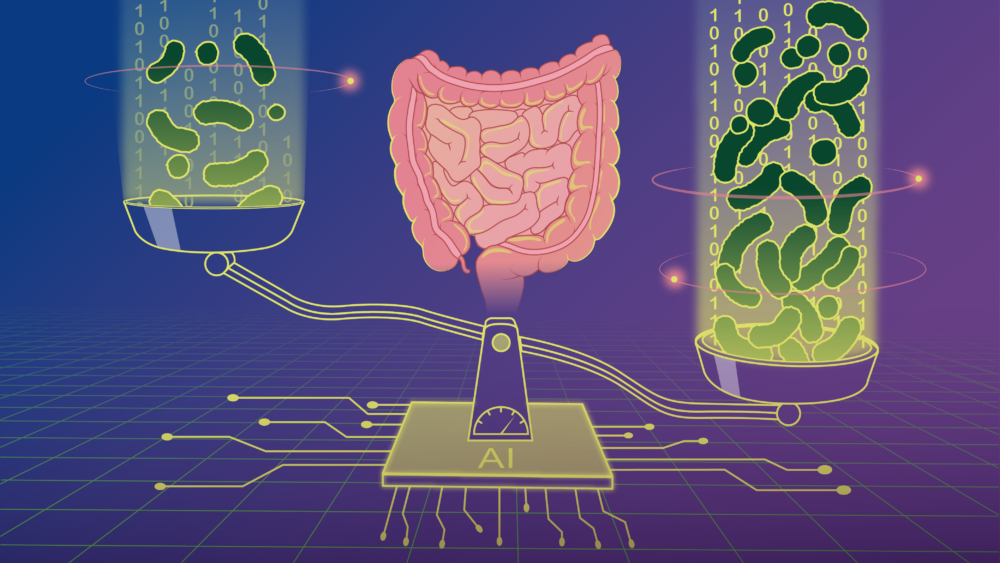
Scientists have developed a new machine-learning model to predict microbial load — the density of microbes in our guts — and used it to demonstrate how microbial load plays an important role in disease-microbiome associations
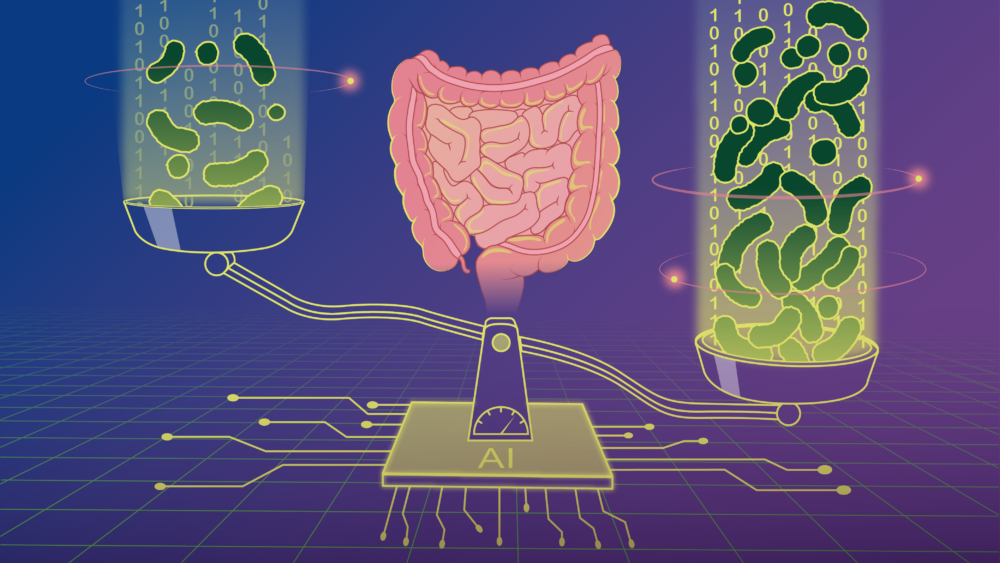
Summary
- Studies on the association between certain diseases and the gut microbiome tend to focus on microbial composition – the relative proportion of different species of microbes in a sample.
- A new study from EMBL Heidelberg demonstrates a machine learning tool to predict microbial load – the density of microbes in a gut microbial sample – from sequencing data without the need for costly additional experiments.
- The study asserts that microbial load is an important factor to consider in disease association studies to avoid false associations or conclusions.
In sickness or in health, the billions of microorganisms that inhabit our guts are our constant companions throughout life. In the past few decades, scientists have shown how the nature of this ‘microbiome’ can provide valuable clues to human diseases and their treatment.
A new study from the Bork group at EMBL Heidelberg, recently published in the journal Cell, reports that a number of conditions, such as lifestyle and disease, affect the total number of microbes in the gut, making this often neglected metric one that bears further evaluation in gut microbiome research.
From composition to loads
When studying microbiomes, researchers tend to focus more strongly on microbial composition – the relative proportion of different species of microbes (usually bacteria and archaea, but also protists, viruses, and other microorganisms). This tells us, for example, whether the level of one species of bacteria goes up or down compared to other species in the guts of certain disease patients.
To illustrate this, imagine that only 1,000 bacteria live in your gut. In healthy individuals, this might include 10 bacteria of species ‘red’, and 20 bacteria of species ‘blue’, so we could say red bacteria make up 2% of the microbiome while blue bacteria make up 5%. However, in individuals who have a particular disease, we might notice red bacteria make up 4% of the microbiome – a relative increase, while blue bacteria remain at 5%. We could then hypothesise that the red bacteria are associated with this disease.
On the other hand, the microbial load refers to the density of microbes inside our guts. Experimentally, it is determined as the number of microbial cells per gram of faeces. Unlike microbial composition, it is an absolute quantity. In the example above, imagine the total number of bacteria dropping to 500 as a result of disease. Looking at the absolute numbers, it’s possible that the number of red bacteria actually stayed the same while the number of blue bacteria decreased.
Scientists usually only consider microbial composition when carrying out microbiome studies because current experimental methods for measuring microbial loads are both time- and cost-intensive.
Using machine learning to make microbiome studies more robust
“We wanted to develop a new method that required no additional experimental methods to quantify microbial load,” said Suguru Nishijima, the study’s first author and a postdoc in the Bork Group. “We had access to large datasets with both microbial composition and experimentally measured microbial load data. We wanted to see if we could use these to train a machine learning model to estimate microbial load given microbial composition alone.”
The datasets used for this exercise came from GALAXY/MicrobLiver and the Metacardis consortia – large-scale EU-funded projects the Bork Group has previously contributed to. Drawn from over 3,700 individuals, these data provided an ideal way to test whether a machine-learning model could be trained to estimate the total number of microbes in a sample.
And indeed, the model created by Nishijima and his colleagues could robustly predict microbial loads, which they validated using a new dataset that the model hadn’t encountered before. Knowing that the model worked, the researchers then applied it to a huge sample of over 27,000 individuals – gathered from 159 previous studies conducted across 45 countries.
They found that many factors can influence microbial load. For example, diarrhoea can reduce the number of microbes in the gut, while constipation can increase them. Women have, on average, a higher microbial load than men (perhaps linked to the observation that women often experience constipation more frequently than men), while young people have a smaller average microbial load than elderly people. Many diseases, as well as the drugs used to treat them, significantly alter microbial load.
“Importantly, many microbial species previously thought to be associated with disease were more strongly explained by variations in microbial load. These findings suggest that changes in microbial load, rather than the disease itself, may be the driver of shifts in the microbiome in patients,” said Nishijima. “However, certain disease-microbe associations remained, and this shows that these are truly robust. This further confirms the importance of including microbial load in microbiome association studies to avoid false positives or false negatives.”
Thanks to the new machine learning model these scientists developed – the first to predict microbial loads from composition data – scientists can now include this important factor in future gut microbiome studies. The model is freely and openly available to researchers worldwide to test and reuse.
This may also have implications far beyond the gut microbiome.
“Our oceans, soils, rivers – are all teeming with microbes, and understanding these microbiomes could yield valuable insights to help preserve our planetary health,” said Peer Bork, Group Leader and Director at EMBL Heidelberg and the study’s senior author. “This study shows us that microbial load is an important measure that must be taken into account in such studies. Thus, we will work towards translating the knowledge on the gut microbiome to other habitats.”
Source article(s)
Fecal microbial load is a major determinant of gut microbiome variation and a confounder for disease associations.
Nishijima S., et al.
Cell 13 December 2024
10.1016/j.cell.2024.10.022